The Rise of Small Language Models (SLMs): The Future of AI is Small, Smart, and Efficient
Meta Description: Discover the surprising advantages of small language models (SLMs) over large language models (LLMs) in AI. Learn about their efficiency, cost-effectiveness, and diverse applications from industry leaders like Microsoft, Nvidia, and OpenAI. Explore the future of AI collaboration between LLMs and SLMs. #SmallLanguageModels #SLMs #AI #ArtificialIntelligence #MachineLearning #EdgeComputing #Efficiency #CostEffective
The AI landscape is changing, and it's not just getting bigger. While giants like Google and OpenAI have been pouring resources into ever-larger, more expensive Large Language Models (LLMs), a quiet revolution is brewing: the rise of Small Language Models (SLMs). Think of LLMs as the heavyweight champions of AI, capable of feats of incredible complexity. But what if you need a nimble, efficient fighter for a specific task? That's where SLMs step into the ring, proving that sometimes, smaller is better. This isn't a case of one technology supplanting the other; rather, it's about synergy - a powerful partnership where each model plays to its strengths. We're witnessing a shift towards a more nuanced, distributed AI ecosystem, one where efficiency and specialized skills are highly valued. This isn’t just a trend; it's a paradigm shift driven by the limitations of colossal LLMs and the exciting potential of their smaller, more adaptable counterparts. From the cost savings in energy consumption to the enhanced security of on-device processing, SLMs are poised to redefine how we interact with artificial intelligence. This article delves into the exciting world of SLMs, exploring their capabilities, advantages, and the collaborative future they promise. Buckle up, because the future of AI is surprisingly compact!
Small Language Models: The Underdogs That Are Changing the Game
For years, the AI race has been a relentless pursuit of bigger and better models. We've seen the awe-inspiring capabilities of models like GPT-4, boasting trillions of parameters. However, this "bigger is better" philosophy isn't always the winning strategy. Enter the SLM, a lean, mean, AI machine designed for specific tasks, offering a compelling alternative to the resource-hungry behemoths. These models typically range from hundreds of millions to a few billion parameters – a significant drop from the gargantuan size of LLMs. This seemingly small difference translates into massive advantages in terms of cost, speed, and energy efficiency.
Imagine needing an AI to analyze customer behavior for a targeted advertising campaign. Using GPT-4 would be like using a sledgehammer to crack a nut – overkill and incredibly expensive. An SLM, on the other hand, would be perfectly suited for the job, providing the necessary insights without the exorbitant resource demands.
This isn't just theoretical; several tech giants are already embracing this change. Microsoft, for instance, has released several SLMs, including the Phi-2 and Phi-3 series, highlighting the growing interest in this technology. Nvidia's Nemotron-Mini-4B-Instruct demonstrates a commitment to providing SLMs optimized for edge computing, emphasizing the potential for deployment in resource-constrained environments. Even OpenAI, the creators of GPT, have recognized the value of SLMs, releasing their own smaller models like GPT-4o mini. These moves clearly indicate a broader industry shift toward recognizing the enormous potential of SLMs.
The Advantages of Small Language Models: Speed, Efficiency, and Cost-Effectiveness
The benefits of SLMs are numerous and compelling:
-
Speed and Responsiveness: SLMs process information significantly faster than their larger counterparts. This makes them ideal for real-time applications where immediate responses are critical, such as chatbots, virtual assistants, and interactive systems. Think instant answers instead of prolonged waits!
-
Reduced Computational Costs: Training and deploying LLMs require vast computational resources, leading to high costs. SLMs, with their smaller size, drastically reduce these costs, making AI accessible to a wider range of businesses and developers. It's a game-changer for startups and smaller companies!
-
Lower Energy Consumption: The energy footprint of LLMs is substantial. SLMs, being more energy-efficient, contribute to a greener AI ecosystem, reducing the environmental impact of AI development and deployment. This is a win-win for the planet and your bottom line!
-
Enhanced Security and Privacy: Because SLMs can be deployed on edge devices, they minimize the need to transfer data to centralized servers. This enhances data security and privacy, addressing a critical concern in AI applications. No more worries about data breaches!
-
Specialized Expertise: While LLMs aim for general-purpose intelligence, SLMs can be trained to excel in niche areas, providing highly accurate and efficient solutions for specific tasks. Think of them as highly skilled specialists focused on one thing.
Microsoft's Phi Series: A Case Study in SLM Innovation
Microsoft's commitment to SLMs is evident in its Phi series of models. Phi-2, with its 2.7 billion parameters, is a remarkable example of powerful yet lightweight AI. Its ability to run on laptops and mobile devices expands the reach of AI significantly. The subsequent Phi-3 and Phi-3.5-mini-instruct models further refine this approach, demonstrating Microsoft's dedication to developing SLMs that cater to diverse computational capabilities and specific tasks. This strategic investment showcases the company's belief in the potential of SLMs to revolutionize AI applications.
The Future of AI: Collaboration Between LLMs and SLMs
The future of AI isn't about choosing between LLMs and SLMs; it's about leveraging the strengths of both. Imagine a system where an SLM acts as a first responder, quickly analyzing a user's request. If the query is complex, the SLM can then pass the information to a larger LLM for in-depth processing. This collaborative approach combines the speed and efficiency of SLMs with the comprehensive capabilities of LLMs, creating a truly powerful and versatile AI system. It's a tag-team approach to problem-solving where speed and power work together.
Edge Computing and the Rise of SLMs
The rise of edge computing is intrinsically linked to the success of SLMs. Edge computing processes data closer to its source, reducing latency and bandwidth requirements. This makes SLMs incredibly well-suited for edge applications, enabling real-time analysis and decision-making without relying on cloud-based infrastructure. This approach not only speeds up processing but also enhances data security and reduces dependency on centralized servers. This is particularly relevant in industries like healthcare, manufacturing, and autonomous vehicles, where real-time processing is crucial.
Frequently Asked Questions (FAQs)
Q1: What exactly is a parameter in an AI model?
A1: A parameter is a value that the AI model learns during training. It allows the model to adapt to the input data and make predictions. More parameters generally mean a more complex model, but not necessarily a better one.
Q2: Are SLMs less accurate than LLMs?
A2: Accuracy depends on the task and the training data. While LLMs might excel at complex tasks requiring vast amounts of information, SLMs can often achieve comparable or even superior accuracy on specific, well-defined tasks with significantly less computational overhead.
Q3: How do SLMs compare to LLMs in terms of energy consumption?
A3: SLMs consume significantly less energy than LLMs. Their smaller size and simpler architecture translate into reduced computational demands, leading to lower energy consumption.
Q4: What are some real-world applications of SLMs?
A4: SLMs are finding applications in various fields, including chatbots, virtual assistants, mobile device AI, edge computing, and specialized tasks in areas like medical image analysis and targeted advertising.
Q5: What is the future of SLMs?
A5: The future is bright! We anticipate further advancements in SLM architecture, leading to even more efficient and powerful models. The integration of SLMs into edge devices and their collaborative work with LLMs will continue to shape the landscape of AI.
Q6: How can I get started learning more about SLMs?
A6: Start by exploring the research papers and publications from leading AI research institutions and companies like Microsoft, Nvidia, and OpenAI. Many resources are freely available online.
Conclusion: A Smaller, Smarter Future for AI
The rise of SLMs marks a significant turning point in the AI journey. While LLMs have their place in tackling complex, data-intensive tasks, SLMs offer a compelling alternative for specific applications where efficiency, cost-effectiveness, and speed are paramount. The future of AI is likely to be a collaborative one, where LLMs and SLMs work together to provide a more powerful and versatile AI experience. The shift towards smaller, smarter AI is not just a trend but a fundamental change in the way we design, deploy, and interact with artificial intelligence. The future of AI is not just bigger, it’s also smaller, smarter, and far more efficient. And that’s a pretty amazing prospect.
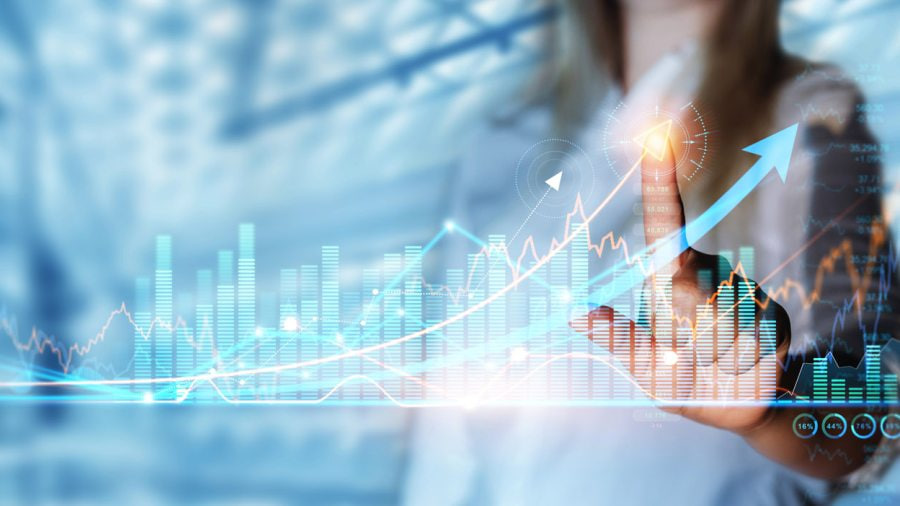